Cellular noise
Cellular noise is random variability in quantities arising in cellular biology. For example, cells which are genetically identical, even within the same tissue, are often observed to have different expression levels of proteins, different sizes and structures.[1][2] These apparently random differences can have important biological and medical consequences.[3]
Cellular noise was originally, and is still often, examined in the context of gene expression levels – either the concentration or copy number of the products of genes within and between cells. As gene expression levels are responsible for many fundamental properties in cellular biology, including cells' physical appearance, behaviour in response to stimuli, and ability to process information and control internal processes, the presence of noise in gene expression has profound implications for many processes in cellular biology.
Definitions
The most frequent quantitative definition of noise is the coefficient of variation:
where is the noise in a quantity , is the mean value of and is the standard deviation of . This measure is dimensionless, allowing a relative comparison of the importance of noise, without necessitating knowledge of the absolute mean.
Another quantity often used for mathematical convenience is the Fano factor:
Intrinsic and extrinsic noise
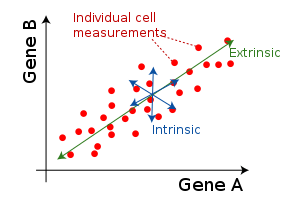
Cellular noise is often investigated in the framework of intrinsic and extrinsic noise. Intrinsic noise refers to variation in identically-regulated quantities within a single cell: for example, the intra-cell variation in expression levels of two identically-controlled genes. Extrinsic noise refers to variation in identically-regulated quantities between different cells: for example, the cell-to-cell variation in expression of a given gene.
Intrinsic and extrinsic noise levels are often compared in dual reporter studies, in which the expression levels of two identically-regulated genes (often fluorescent reporters like GFP and YFP) are plotted for each cell in a population.[4]
Sources
Note: These lists are illustrative, not exhaustive, and identification of noise sources is an active and expanding area of research.
- Intrinsic noise
- Low copy-number effects (including discrete birth and death events): the random nature of production and degradation of cellular components means that noise is high for components at low copy number (as the magnitude of these random fluctuations is not negligible with respect to the copy number);
- Diffusive cellular dynamics:[5] many important cellular processes rely on collisions between reactants (for example, RNA polymerase and DNA) and other physical criteria which, given the diffusive dynamic nature of the cell, occur stochastically.
- Noise propagation: Low copy-number effects and diffusive dynamics result in each of the biochemical reactions in a cell occurring randomly. Stochasticity of reactions can be either attenuated or amplified. Contribution each reaction makes to the intrinsic variability in copy numbers can be quantified via Van Kampen's system size expansion.[6][7]
- Extrinsic noise
- Cellular age / cell cycle stage: cells in a dividing population that is not synchronised will, at a given snapshot in time, be at different cell cycle stages, with corresponding biochemical and physical differences;[8]
- Physical environment (temperature, pressure, ...): physical quantities and chemical concentrations (particularly in the case of cell-to-cell signalling) may vary spatially across a population of cells, provoking extrinsic differences as a function of position;[9]
- Organelle distributions: random factors in the quantity and quality of organelles (for example, the number and functionality of mitochondria[10]) lead to significant cell-to-cell differences in a range of processes[11] (as, for example, mitochondria play a central role in the energy budget of eukaryotic cells);
- Inheritance noise: uneven partitioning of cellular components between daughter cells at mitosis can result in large extrinsic differences in a dividing population.[12]
Note that extrinsic noise can affect levels and types of intrinsic noise:[13] for example, extrinsic differences in the mitochondrial content of cells lead, through differences in ATP levels, to some cells transcribing faster than others, affecting the rates of gene expression and the magnitude of intrinsic noise across the population.[11]
Effects
Note: These lists are illustrative, not exhaustive, and identification of noise effects is an active and expanding area of research.
- Gene expression levels: noise in gene expression causes differences in the fundamental properties of cells, limits their ability to biochemically control cellular dynamics,[14] and directly or indirectly induce many of the specific effects below;
- Energy levels and transcription rate: noise in transcription rate, arising from sources including transcriptional bursting, is a significant source of noise in expression levels of genes. Extrinsic noise in mitochondrial content has been suggested to propagate to differences in the ATP concentrations and transcription rates (with functional relationships implied between these three quantities) in cells, affecting cells' energetic competence and ability to express genes;[11]
- Phenotype selection: bacterial populations exploit extrinsic noise to choose a population subset to enter a quiescent state.[15] In a bacterial infection, for example, this subset will not propagate quickly but will be more robust when the population is threatened by antibiotic treatment: the rapidly replicating, infectious bacteria will be killed more quickly than the quiescent subset, which may be capable of restarting the infection. This phenomenon is why courses of antibiotics should be finished even when symptoms seem to have disappeared;
- Development and stem cell differentiation: developmental noise in biochemical processes which need to be tightly controlled (for example, patterning of gene expression levels that develop into different body parts) during organismal development can have dramatic consequences, necessitating the evolution of robust cellular machinery. Stem cells differentiate into different cell types depending on the expression levels of various characteristic genes:[16] noise in gene expression can clearly perturb and influence this process, and noise in transcription rate can affect the structure of the dynamic landscape that differentiation occurs on;[11]
- Cancer treatments: recent work has found extrinsic differences, linked to gene expression levels, in the response of cancer cells to anti-cancer treatments, potentially linking the phenomenon of fractional killing (whereby each treatment kills some but not all of a tumour) to noise in gene expression.[17] Because individual cells could repeatedly and stochastically perform transitions between states associated with differences in responsiveness to a therapeutic modality (chemotherapy, targeted agent, radiation, etc.), therapy might need to be administered frequently (to ensure cells are treated soon after entering a therapy-responsive state, before they can rejoin the therapy-resistant subpopulation and proliferate) and over long times (to treat even those cells emerging late from the final residue of the therapy-resistant subpopulation).[18]
- Information processing: as cellular regulation is performed with components that are themselves subject to noise, the ability of cells to process information and perform control is fundamentally limited by intrinsic noise[14][19]
Analysis
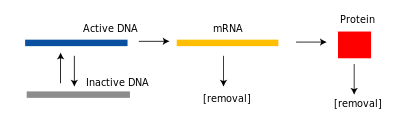
As many quantities of cell biological interest are present in discrete copy number within the cell (single DNAs, dozens of mRNAs, hundreds of proteins), tools from discrete stochastic mathematics are often used to analyse and model cellular noise.[20][21] In particular, master equation treatments – where the probabilities of observing a system in a state at time are linked through ODEs – have proved particularly fruitful. A canonical model for noise gene expression, where the processes of DNA activation, transcription and translation are all represented as Poisson processes with given rates, gives a master equation which may be solved exactly (with generating functions) under various assumptions or approximated with stochastic tools like Van Kampen's system size expansion.
Numerically, the Gillespie algorithm or stochastic simulation algorithm is often used to create realisations of stochastic cellular processes, from which statistics can be calculated.
The problem of inferring the values of parameters in stochastic models (parametric inference) for biological processes, which are typically characterised by sparse and noisy experimental data, is an active field of research, with methods including Bayesian MCMC and approximate Bayesian computation proving adaptable and robust.
References
- ↑ Kaern, M.; Elston, T.R.; Blake, W.J. & Collins, J.J. (2005). "Stochasticity in gene expression: from theories to phenotypes". Nat. Rev. Genet. 6: 451–464. doi:10.1038/nrg1615.
- ↑ Maheshri N, O'Shea EK (2007). "Living with noisy genes: how cells function reliably with inherent variability in gene expression". Annu. Rev. Biophys. Biomol. Struct. 36: 413–434. doi:10.1146/annurev.biophys.36.040306.132705.
- ↑ Johnston, I. G. (2012). "The chaos within: exploring noise in cellular biology". Significance. 19 (4): 17–21. doi:10.1111/j.1740-9713.2012.00586.x.
- ↑ Elowitz, M.B.; Levine, A.J.; Siggia, E.D.; Swain, P.S. (2002). "Stochastic gene expression in a single cell". Science. 297 (5584): 1183–6. PMID 12183631. doi:10.1126/science.1070919.
- ↑ Morelli, M.J.; Allen, R.J. & ten Wolde, P.R. (2011). "Effects of macromolecular crowding on genetic networks". Biophys. J. 101: 2882–2891. PMC 3244068
. PMID 22208186. doi:10.1016/j.bpj.2011.10.053.
- ↑ Komorowski M, Miekisz J & Stumpf M PH (2013). "Decomposing Noise in Biochemical Signaling Systems Highlights the Role of Protein Degradation". Biophys. J. 104: 1783–1793. PMC 3627874
. PMID 23601325. doi:10.1016/j.bpj.2013.02.027.
- ↑ Jetka T, Charzynska A, Gambin A, Stumpf M PH & Komorowski M (2013). "StochDecomp - Matlab package for noise decomposition in stochastic biochemical systems". Bioinformatics. 30: 137–138. PMID 24191070. doi:10.1093/bioinformatics/btt631.
- ↑ Newman JR, Ghaemmaghami S, Ihmels J, Breslow DK, Noble M, DeRisi JL, Weissman JS (2006). "Single-cell proteomic analysis of S. cerevisiae reveals the architecture of biological noise". Nature. 441: 840–846. PMID 16699522. doi:10.1038/nature04785.
- ↑ Athale, C.A.; Chaudhari, H. (2011). "Population length variability and nucleoid numbers in Escherichia coli". Bioinformatics. 27: 2944–2998. PMID 21930671. doi:10.1093/bioinformatics/btr501.
- ↑ das Neves RP, Jones NS, Andreu L, Gupta R, Enver T, Iborra FJ (2010). "Connecting Variability in Global Transcription Rate to Mitochondrial Variability". PLoS Biol. 8 (12): e1000560. PMC 3001896
. PMID 21179497. doi:10.1371/journal.pbio.1000560.
- 1 2 3 4 Johnston IG, Gaal B, das Neves RP, Enver T, Iborra FJ, Jones NS (2012). "Mitochondrial Variability as a Source of Extrinsic Cellular Noise". PLoS Comput. Biol. 8 (3): e1002416. PMC 3297557
. PMID 22412363. doi:10.1371/journal.pcbi.1002416.
- ↑ Huh, D.; Paulsson, J. (2011). "Random partitioning of molecules at cell division". Proc. Natl. Acad. Sci. USA. 108 (36): 15004–15009. doi:10.1073/pnas.1013171108.
- ↑ Shahrezaei, V. & Swain, P.S. (2008). "Analytical distributions for stochastic gene expression". Proc. Natl. Acad. Sci. USA. 105 (45): 17256–17261. doi:10.1073/pnas.0803850105.
- 1 2 Lestas, I.; Vinnicombe, G.; Paulsson, J. (2010). "Fundamental limits on the suppression of molecular fluctuations". Nature. 467 (7312): 174–8. PMC 2996232
. PMID 20829788. doi:10.1038/nature09333.
- ↑ Fraser D, Kaern M (2009). "A chance at survival: gene expression noise and phenotypic diversification strategies.". Mol. Microbiol. 71: 1333–1340. PMID 19220745. doi:10.1111/j.1365-2958.2009.06605.x.
- ↑ T. Enver; C.M. Heyworth & T.M. Dexter (1998). "Do Stem Cells Play Dice?". Blood. 92: 348.
- ↑ Spencer, S.L.; Gaudet, S.; Albeck, J.G.; Burke, J.M.; Sorger, P.K. (2009). "Non-genetic origins of cell-to-cell variability in TRAIL-induced apoptosis". Nature. 459 (7245): 428–432. doi:10.1038/nature08012.
- ↑ Liao D, Estévez-Salmerón L & Tlsty TD (2012). "Conceptualizing a tool to optimize therapy based on dynamic heterogeneity". Phys. Biol. 9: 065005. doi:10.1088/1478-3975/9/6/065005.
- ↑ Perkins, T.J. & Swain, P.S. (2009). "Strategies for cellular decision-making". Mol. Sys. Bio. 5 (236). doi:10.1038/msb.2009.83.
- ↑ Paulsson, J. (2005). "Models of stochastic gene expression". Phys. Life Rev. 2 (2): 157–175. doi:10.1016/j.plrev.2005.03.003.
- ↑ Wilkinson, D.J. (2009). "Stochastic modelling for quantitative description of heterogeneous biological systems". Nat. Rev. Genet. 10 (2): 122–133. PMID 19139763. doi:10.1038/nrg2509.