Targeted projection pursuit
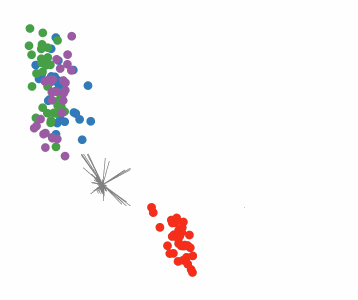
Targeted projection pursuit is a type of statistical technique used for exploratory data analysis, information visualization, and feature selection. It allows the user to interactively explore very complex data (typically having tens to hundreds of attributes) to find features or patterns of potential interest.
Conventional, or 'blind', projection pursuit, finds the most "interesting" possible projections in multidimensional data, using a search algorithm that optimizes some fixed criterion of "interestingness" – such as deviation from a normal distribution. In contrast, targeted projection pursuit allows the user to explore the space of projections by manipulating data points directly in an interactive scatter plot.
Targeted projection pursuit has found applications in DNA microarray data analysis,[1] protein sequence analysis,[2] graph layout[3] and digital signal processing.[4] It is available as a package for the WEKA machine learning toolkit.
References
- ↑ Faith, Joseph; Robert Mintram; Maia Angelova (2006). "Targeted Projection Pursuit for Visualising Gene Expression Data Classifications" (PDF). Bioinformatics 22 (21): 2667–267. doi:10.1093/bioinformatics/btl463.
- ↑ Haddow, Chris; Marcus Durrant; Justin Perry; Joe Faith (in press). "Predicting Functional Residues of Protein Sequence Alignments as a Feature Selection Task". International Journal of Data Mining in Bioinformatics. Check date values in:
|date=
(help) - ↑ Gibson, Helen; Joe Faith (2011). "Node-Attribute Graph Layout for Small-World Networks". Proceedings of 15th International Conference on Information Visualisation.
- ↑ Sujan, Rajbhandari; Joe Faith (2010). "The Use of Linear Projections in the Visual Analysis of Signals in an Indoor Optical Wireless Link". IEEE, IET International Symposium on Communication Systems, Networks and Digital Signal Processing.
Further reading
- Joe Faith (2007) "Targeted Projection Pursuit for Interactive Exploration of High-Dimensional Data Sets", Proceedings of 11th International Conference on Information Visualisation
External links
- imDEV free Excel add-in for targeted projection pursuits using feature selection coupled with PLS and PLS-DA
- Targeted Projection Pursuit project page