Cohort analysis
Cohort analysis is a subset of behavioral analytics that takes the data from a given eCommerce platform, web application, or online game and rather than looking at all users as one unit, it breaks them into related groups for analysis. These related groups, or cohorts, usually share common characteristics or experiences within a defined time-span. Cohort analysis allows a company to “see patterns clearly across the life-cycle of a customer (or user), rather than slicing across all customers blindly without accounting for the natural cycle that a customer undergoes.”[1] By seeing these patterns of time, a company can adapt and tailor its service to those specific cohorts. While cohort analysis is sometimes associated with a cohort study, they are different and should not be viewed as one and the same. Cohort analysis has come to describe specifically the analysis of cohorts in regards to big data and business analytics, while a cohort study is a more general umbrella term that describes a type of study in which data is broken down into similar groups.
Examples
The goal of any business analytic tool is to analyze and present actionable information to a company to act on, and focus on the "average user" of a given system.[2] In order for a company to act on such info it must be relevant to the situation at hand. A database full of thousands or even millions of entries of all user data makes it tough to gain actionable data, as those data span many different categories and time periods. Actionable cohort analysis allows for the ability to drill down to the users of each specific cohort to gain a better understanding of their behaviors, such as if users checked out, and how much did they pay. In cohort analysis "each new group [cohort] provides the opportunity to start with a fresh set of users,"[3] allowing the company to look at only the data that is relevant to the current query and act on it.
In eCommerce, a firm may only be interested in customers who signed up in the last two weeks and who made a purchase, which is an example of a specific cohort. A software developer may only care about the data from users who sign up after a certain upgrade, or who use certain features of the platform.
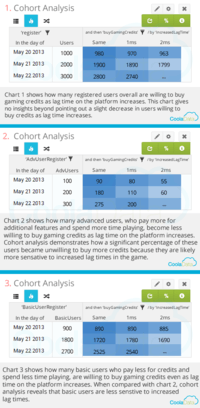
An example of cohort analysis of gamers on a certain platform: Expert gamers, cohort 1, will care more about advanced features and lag time compared to new sign-ups, cohort 2. With these two cohorts determined, and the analysis run, the gaming company would be presented with a visual representation of the data specific to the two cohorts. It could then see that a slight lag in load times has been translating into a significant loss of revenue from advanced gamers, while new sign-ups have not even noticed the lag. Had the company simply looked at its overall revenue reports for all customers, it would not have been able to see the differences between these two cohorts. Cohort analysis allows a company to pick up on patterns and trends and make the changes necessary to keep both advanced and new gamers happy.
Deep actionable cohort analytics
"An actionable metric is one that ties specific and repeatable actions to observed results [like user registration, or checkout]. The opposite of actionable metrics are vanity metrics (like web hits or number of downloads) which only serve to document the current state of the product but offer no insight into how we got here or what to do next."[4] Without actionable analytics the information that is being presented may not have any practical application, as the only data points represent vanity metrics that do not translate into any specific outcome. While it is useful for a company to know how many people are on their site, that metric is useless on its own. For it to be actionable it needs to relate a "repeatable action to [an] observed result".[4]
Performing cohort analysis
In order to perform a proper cohort analysis, there are four main stages:[5]
- Determine what question you want to answer- The point of the analysis is to come up with some actionable information for a company to act on and improve its business, product, user experience, turnover... To make sure that this happens it is important that the right question is asked so that the correct solution is found. In the gaming example above, the company was unsure why they were losing revenue as lag time increased despite the fact that users were still signing up and playing games.
- Define the metrics that will be able to help you answer the question- A proper cohort analysis requires the identification of the specific properties of an event. Such an event might include a record of a user checking out, while more advanced metrics will not only record a checkout, but also how much did the user pay. The gaming example measured a customers willingness to buy gaming credits based on how much lag time there was on the site.
- Define the specific cohorts that are relevant- In creating a cohort one must analyze all the actual users and target them, or perform attribute contribution in order to find the relevant differences between each of them, in order to find out and explain their behavior as a specific cohort. The above example splits users into "basic" and "advanced" users as each group acts differently and has different pricing structure sensitivities, and usage levels.
- Perform the cohort analysis- The analysis in the above example was done through the use of visual graphs. This visualization allowed the gaming company to realize that their revenues were falling because their higher paying "advanced" users were not using the system as the lag time increased. Since they were such a large portion of the companies revenue, the additional "basic" users who were signing up were not covering the losses from the "advanced users. In order to fix this the company improved their lag times and began catering more to their "advanced" users.
See also
References
- ↑ Alistair Croll; Benjamin Yoskovitz. Lean Analytics: Use Data to Build a Better Startup Faster. Sebastopol, CA: O'Reilly. ISBN 1449335675.
- ↑ Aukeman, Mark. "Cohort Analysis — understanding your customers". edwblog.com.
- ↑ Balogh, Jonathon. "Introduction to Cohort Analysis for Startups".
- 1 2 Maurya, Ash. "3 Rules to Actionable Metrics in a Lean Startup".
- ↑ James Torio; Rishabh Dayal. "Using Cohort Analysis to Optimize Customer Experience". UX Magazine.
Further reading
- "Cohort Analysis with Google Analytics". Analytics Talk. Justin Cutroni.
- "Get Useful Insights Easier: Automate Cohort Analysis with Analytics & Tableau". Google Analytics Blog. Google.
- Glenn, Norval D. (2005). Cohort Analysis. Quantitative Applications in the Social Sciences vol. 07-005 (2nd ed.). Sage Publication.
- Wilson, Fred. "The Cohort Analysis". AVC.
- PORTER, JOSHUA. "COHORT ANALYSIS - MEASURING ENGAGEMENT OVER TIME". 52 Weeks of UX.
- "Intro Guide to Cohort Analysis". Insights Blog. Canopy Labs.