Balanced histogram thresholding
In image processing, the balanced histogram thresholding method (BHT),[1] is a very simple method used for automatic image thresholding. Like Otsu's Method[2] and the Iterative Selection Thesholding Method,[3] this is a histogram based thresholding method. This approach assumes that the image is divided in two main classes: The background and the foreground. The BHT method tries to find the optimum threshold level that divides the histogram in two classes.
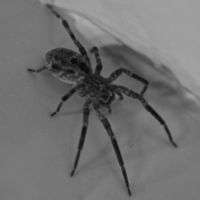
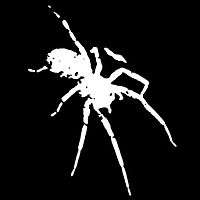
This method weighs the histogram, checks which of the two sides is heavier, and removes weight from the heavier side until it becomes the lighter. It repeats the same operation until the edges of the weighing scale meet.
Given its simplicity, this method is a good choice as a first approach when presenting the subject of automatic image thresholding.
Algorithm
The following listing, in C notation, is a simplified version of the Balanced Histogram Thresholding method:
int BHThreshold(int[] histogram) {
i_m = (int)((i_s + i_e) / 2.0f); // center of the weighing scale I_m
w_l = get_weight(i_s, i_m + 1, histogram); // weight on the left W_l
w_r = get_weight(i_m + 1, i_e + 1, histogram); // weight on the right W_r
while (i_s <= i_e) {
if (w_r > w_l) { // right side is heavier
w_r -= histogram[i_e--];
if (((i_s + i_e) / 2) < i_m) {
w_r += histogram[i_m];
w_l -= histogram[i_m--];
}
} else if (w_l >= w_r) { // left side is heavier
w_l -= histogram[i_s++];
if (((i_s + i_e) / 2) >= i_m) {
w_l += histogram[i_m + 1];
w_r -= histogram[i_m + 1];
i_m++;
}
}
}
return i_m;
}
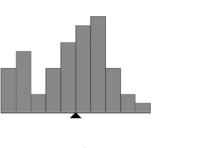
This method may have problems when dealing with very noisy images, because the weighing scale may be misplaced. The problem can be minimized by ignoring the extremities of the histogram.[4]
References
- ↑ A. Anjos and H. Shahbazkia. Bi-Level Image Thresholding - A Fast Method. BIOSIGNALS 2008. Vol:2. P:70-76.
- ↑ Nobuyuki Otsu (1979). "A threshold selection method from gray-level histograms". IEEE Trans. Sys., Man., Cyber. 9: 62–66.
- ↑ Ridler TW, Calvard S. (1978) Picture thresholding using an iterative selection method, IEEE Trans. System, Man and Cybernetics, SMC-8: 630-632.
- ↑ A. Anjos, R. Leite, M. L. Cancela, H. Shahbazkia. MAQ – A Bioinformatics Tool for Automatic Macroarray Analysis. International Journal of Computer Applications. 2010. Number 7 - Article 1.