Neuronal noise
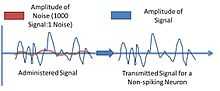
Neuronal noise or neural noise refers to the random intrinsic electrical fluctuations within neuronal networks. These fluctuations are not associated with encoding a response to internal or external stimuli and can be from one to two orders of magnitude.[1] Most noise commonly occurs below a voltage-threshold that is needed for an action potential to occur, but sometimes it can be present in the form of an action potential; for example, stochastic oscillations in pacemaker neurons in suprachiasmatic nucleus are partially responsible for the organization of circadian rhythms.[2][3]
Background
Neuronal noise begins at the microscopic level with atomic collisions and agitation.[4] On the postsynaptic membrane, neural noise has been evident in the early stages of processing sight, smell, and hearing. The exact reason for neuronal noise relating to computational processing is still not currently understood, but a great deal of theories have been proposed.
Single neurons demonstrate different responses to specific neuronal input signals. This is commonly referred to as neural response variability. If a specific input signal is initiated in the dendrites of a neuron, then a hypervariability exists in the number of vesicles released from the axon terminal fiber into the synapse.[5] This characteristic is true for fibers without neural input signals, such as pacemaker neurons, as mentioned previously,[2] and cortical pyramidal neurons that have highly-irregular firing pattern.[6] Noise generally hinders neural performance, but recent studies show, in dynamical non-linear neural networks, this statement does not always hold true. Non-linear neural networks are a network of complex neurons that have many connections with one another such as the neuronal systems found within our brains. Comparatively, linear networks are an experimental view of analyzing a neural system by placing neurons in series with each other.
Initially, noise in complex computer circuit or neural circuits is thought to slow down[7] and negatively affect the processing power. However, current research suggests that neuronal noise is beneficial to non-linear or complex neural networks up until optimal value.[8] A theory by Anderson and colleagues supports that neural noise is beneficial. Their theory suggests that noise produced in the visual cortex helps linearize or smooth the threshold of action potentials.[9]
Another theory suggests that stochastic noise in a non-linear network shows a positive relationship between the interconnectivity and noise-like activity.[10] Thus based on this theory, Patrick Wilken and colleagues suggest that neuronal noise is the principal factor that limits the capacity of visual short-term memory. Investigators of neural ensembles and those who especially support the theory of distributed processing, propose that large neuronal populations effectively decrease noise by averaging out the noise in individual neurons. Some investigators have shown in experiments and in models that neuronal noise is a possible mechanism to facilitate neuronal processing.[11][12] The presence of neuronal noise (or more specifically synaptic noise) confers to neurons more sensitivity to a broader range of inputs, it can equalize the efficacy of synaptic inputs located at different positions on the neuron, and it can also enable finer temporal discrimination.[13] There are many theories of why noise is apparent in the neuronal networks, but many neurologists are unclear of why they exist.
More generally, two types of impacts of neuronal noise can be distinguished: it will either add variability to the neural response, or more interestingly enable noise - induced dynamical phenomena which would not have been observed in a noise-free system. For instance, channel noise has been shown to induce oscillations in the stochastic Hodgkin-Huxley model.[14]
Types
- Ions exist inside and outside of the neuron and are subject to many bodily conditions. One major source of noise arises from ions or molecules in response to the third law of thermodynamics. This law states that the entropy of a system approaches a constant value as the temperature approaches zero. Since the body maintains temperatures above 0K, the molecules are subjected to increased kinetic energy, or motion. The stochastic, or random, movements give rise to receptor noise produced by the constant bombardment of ions, as described by Brownian motion. Ions are constantly being leaked across the membrane in efforts to equalize the ionic gradient produced by ATPase channels embedded in the membrane. These leaky channels permit the movement of ions across the membrane leading to small fluctuations, or noise, in the membrane potential.[15]
- Synapses are another major source of neural noise. More than often, there is random exocytosis of vesicles containing neurotransmitters, which eventually bind to the postsynaptic membrane, leading to a spontaneous action potential by graded potentials in the postsynaptic neuron.[16] It is considered the largest-amplitude noise source in the cerebral cortex.[4]
Sources
Noise present in neural system gives rise to the variability in the non-linear dynamical systems, but a black box still exists for the mechanism in which noise affects neural signal conduction. Instead, research has focused more on the sources of the noise present in dynamic neural networks. Several sources of response variability exist for neurons and neural networks:[17]
- Thermal noise: Johnson–Nyquist noise occurs due to the thermal motions of ions and other charge carriers, producing voltage fluctuations proportional to temperature. This source of noise is attributed to the third law of thermodynamics, stating that kinetic energy of molecules increases with a raise in temperature. Thermal noise is the weakest source of noise and can be considered negligible.[18]
- Ionic conductance noise: Ion channels in the membrane undergo spontaneous changes in conformation between different states and can open (or close) due to thermal fluctuations. The transmembrane embedded protein channels are made up of small subunits that undergo conformational changes and are affected by thermal fluctuations. When temperature drops below 33°C, the rate at which the channel becomes active or inactive decreases. In contrast when the temperature is increased above 33°C, the rate at which the channel becomes active or inactive increases.[19]
- Ion pump noise: Membrane embedded ATPase ion pumps produce fluctuating potentials by transporting ions against their concentration gradient. The multistep process in which ions are transported across their gradient requires ATP.[20] The steps involved in active transport have a net forward direction, but small stochastic steps still exist in the conformational process that move backwards.[20] These backward steps contribute to neuronal noise present in all dynamic neuronal circuits.
- Ion channel shot noise: The number of ions that migrate through an open ion channel are discrete and random.[21] In synapses, the number of calcium ions that enter the postsynaptic side after a spike is on the order of 250 ions,[17] potentially making potentiation processes noisy. This noise is also associated with thermal fluctuations affecting the protein channels, as previously mentioned. This is not to be confused with shot noise, which is noise produced by the random generation of action potentials in neurons.
- Synaptic release noise: Generally, action potentials are transferred down a neuron, which then are converted to either electrical or chemical signals between neurons. Chemical synapses are not deterministic, which means that every action potential produced does not result in the release of neurotransmitters. Rather, the release of vesicles containing neurotransmitters are probabilistic in nature. The number of vesicles released by a single synapse is random in response to a specific input signal and is further influenced by the firing history of the pre- and post-synaptic neurons. This means that neurotransmitters can be released in the absence of an input signal.[17]
- Synaptic bombardment: The large number of incoming spikes add a fluctuating amount of charge to the cell, which depends on the structure of the incoming spike trains and affects the cell's excitability.[22]
- Chaos: Chaotic dynamics can occur in single cells (due to periodic inputs or bursting due to intrinsic currents).[23] Simple networks of neurons can also exhibit chaotic dynamics.[24] Even if the chaos is deterministic, it can amplify noise from the other sources to macroscopic levels due to sensitive dependence on initial conditions.
- Connectivity noise: Noise that arises from the number of connections and non-uniformity that a neuron has with other neurons within a neuronal network. There is a stronger presence of sub-threshold noise when the interconnectivity is strengthened, or the number of connection to other neurons is increased.[10] The opposite remains true, too. If the interconnectivity of the neurons is decreased so then is the level of sub-threshold noise.
- Environmental Stimuli: Noise can be produced on a larger scale due to fluctuations in CO2, which lead to variations in blood flow.[25] The level of CO2 in the blood allows for either vasoconstriction or vasodilation, which can encroach, or expand, into nearby neural networks producing noise.
Recording Methods
Global Recording
The external noise paradigm is used to test the presence or absence of neural noise. According to this paradigm, external noise should multiplicatively increase the amount of internal noise in the central nervous system. Researchers add visual or auditory external noise to a stimuli, and measure how it affects reaction time or the subject's performance. If performance is more inconsistent than without the noise, the subject has internal noise.
- Electroencephalogram or EEG can be used to measure the brain since the signal-to-noise ratio is poor, so noise produced by the brain can be detected in vivo.
- Local Field Potentials can be used to test the noise present in large neuronal networks. This measure of voltage can be used to determine the interconnectivity by the level of noise.[10]
Local Recording
Local recording has contributed a lot to discovering many of the new sources of ion channel noise.
- Patch Clamp technique was crucial to determine shot noise because the use of intercellular recording was not able to show the movement or detection of the few ions escaping at a time.
See also
References
- ↑ Jacobson, G. A., et al. (2005). "Subthreshold voltage noise of rat neocortical pyramidal neurones." J Physiol 564(Pt 1): 145-160.
- ↑ 2.0 2.1 Ko, C. H., et al. (2010). "Emergence of Noise-Induced Oscillations in the Central Circadian Pacemaker." Plos Biology 8(10).
- ↑ Mazzoni, E. O., et al. (2005). "Circadian Pacemaker Neurons Transmit and Modulate Visual Information to Control a Rapid Behavioral Response." Neuron 45(2): 293-300.
- ↑ 4.0 4.1 Destexhe, A. (2012). Neuronal noise. New York: Springer.
- ↑ Stein, R. B., et al. (2005). "Neuronal variability: noise or part of the signal?" Nature Reviews Neuroscience 6(5): 389-397.
- ↑ Softky, W. R. and C. Koch (1993). "The highly irregular firing of cortical cells is inconsistent with temporal integration of random EPSPs." J Neurosci 13(1): 334-350.
- ↑ McDonnell, Mark D., and Lawrence M. Ward. "The Benefits Of Noise In Neural Systems: Bridging Theory And Experiment." Nature Reviews Neuroscience 12.7 (2011): 415-426. Academic Search Complete. Web. 19 Nov. 2012.
- ↑ Parnas, B. R. (1996). "Noise and neuronal populations conspire to encode simple waveforms reliably." IEEE Trans Biomed Eng 43(3): 313-318.
- ↑ Anderson, J. S., et al. (2000). "The contribution of noise to contrast invariance of orientation tuning in cat visual cortex." Science 290(5498): 1968-1972.
- ↑ 10.0 10.1 10.2 Serletis, D., et al. (2011). "Complexity in neuronal noise depends on network interconnectivity." Ann Biomed Eng 39(6): 1768-1778.
- ↑ http://www.rochester.edu/news/show.php?id=2683
- ↑ Ma, W.J., Beck, J., Latham, P. and Pouget, A., Bayesian inference with probabilistic population codes. Nature Neuroscience. 9(11), 1432-1438. 2006. http://www.bcs.rochester.edu/people/alex/pub/articles/MaBeckLathamPougetNN06.pdf
- ↑ See the "High-conductance state" article in Scholarpedia.
- ↑ Wainrib, Gilles; Thieullen, Michèle; Pakdaman, Khashayar (13 August 2011). "Reduction of stochastic conductance-based neuron models with time-scales separation". Journal of Computational Neuroscience 32 (2): 327–346. doi:10.1007/s10827-011-0355-7.
- ↑ Randall, D. J., et al. (2002). Eckert animal physiology : mechanisms and adaptations. New York, W.H. Freeman and Co.
- ↑ Fatt, P. and B. Katz (1952). "Spontaneous subthreshold activity at motor nerve endings." J Physiol 117(1): 109-128.
- ↑ 17.0 17.1 17.2 Christoph Koch, Biophysics of Computation. Oxford University Press, New York, 1999
- ↑ Manwani A, Koch C (1999c) Signal detection in noisy weakly active dendrites. In: Kearns MS, Solla SA, Cohn DA, eds. Advances in Neural Information Processing Systems 11. MIT Press, Cambridge, MA.
- ↑ Cao, X. J. and D. Oertel (2005). "Temperature affects voltage-sensitive conductances differentially in octopus cells of the mammalian cochlear nucleus." J Neurophysiol 94(1): 821-832.
- ↑ 20.0 20.1 Lauger, P. (1984). "Current noise generated by electrogenic ion pumps." Eur Biophys J 11(2): 117-128.
- ↑ Brunetti, R., et al. (2007). "Shot noise in single open ion channels: A computational approach based on atomistic simulations." Journal of Computational Electronics 6(1): 391-394.
- ↑ N Ho and A Destexhe, Synaptic background activity enhances the responsiveness of neocortical pyramidal neurons. J. Neurophysiol. 84, 1488 (2000)
- ↑ A Longtin, Autonomous stochastic resonance in bursting neurons. Phys. Rev. E. 55, 868 (1997)
- ↑ Li, C., Yu, J., & Liao, X. (2001). Chaos in a three‐neuron hysteresis hopfield‐type neural networks. Physics Letters A, 285, 368-372.
- ↑ Birn, R. M. (2012). The role of physiological noise in resting-state functional connectivity. [Review]. Neuroimage, 62(2), 864-870. doi: 10.1016/j.neuroimage.2012.01.016
External links
- Neuronal Noise article in Scholarpedia
- High-Conductance State article in Scholarpedia