Biological clock (aging)
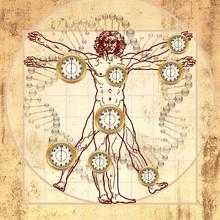
Also known as epigenetic clock, DNA clock, Horvath's clock or DNA methylation age, this biological aging clock is an "age predictor" that can be used to measure the age of most human tissues, cell types, or organs".[1][2][3] Salient features of the epigenetic clock include its high accuracy and its applicability to a broad spectrum of tissues and cell types. Since it allows one to contrast the ages of different tissues from the same subject, it can be used to identify tissues that show evidence of accelerated age due to disease.
Properties of the epigenetic clock
The clock is defined as an age prediction method based on 353 epigenetic markers on the DNA. The 353 markers measure DNA methylation of CpG dinucleotides. Predicted age, referred to as DNA methylation age, has the following properties: first, it is close to zero for embryonic and induced pluripotent stem cells; second, it correlates with cell passage number; third, it gives rise to a highly heritable measure of age acceleration; and, fourth, it is applicable to chimpanzee tissues. Organismal growth (and concomitant cell division) leads to a high ticking rate of the epigenetic clock that slows down to a constant ticking rate (linear dependence) after adulthood (age 20).[1] The fact that DNA methylation age of blood predicts all-cause mortality in later life even after adjusting for known risk factors [4] suggests that it relates to a process that causes aging. Similarly, markers of physical and mental fitness are associated with the epigenetic clock (lower abilities associated with age acceleration).[5]
Motivation of biological clocks
In general, biological aging clocks and biomarkers of aging are expected to find many uses in biological research since age is a fundamental characteristic of most organisms. Accurate measures of biological age (biological aging clocks) could be useful for
- testing the validity of various theories of biological aging,
- diagnosing various age related diseases and for defining cancer subtypes,
- predicting/prognosticating the onset of various diseases,
- serving as surrogate markers for evaluating therapeutic interventions including rejuvenation approaches,
- studying developmental biology and cell differentiation,
- forensic applications, for example to estimate the age of a suspect based on blood left on a crime scence.
Overall, biological clocks are expected to be useful for studying what causes aging and what can be done against it.
History of the epigenetic clock
The epigenetic clock was developed by Steve Horvath, a professor of human genetics at the David Geffen School of Medicine at UCLA and of biostatistics at the UCLA Fielding School of Public Health. The scientific article was first published on Oct 21, 2013 in Genome Biology.[1][2] Horvath spent over 4 years collecting publicly available Illumina DNA methylation data and identifying suitable statistical methods.[6] The personal story behind the discovery was featured in Nature.[7] The age predictor was developed using 8,000 samples from 82 Illumina DNA methylation array datasets, encompassing 51 healthy tissues and cell types. The strong effect of age on DNA methylation levels has been known since the late 1960s.[8] A vast literature describes sets of CpGs whose DNA methylation levels correlate with age, e.g.[9][10][11][12][13] Previous publications describe age predictors based on DNA methylation levels in either saliva [14] or blood.[15] The major innovation of the epigenetic clock lies in its wide applicability: the same set of 353 CpGs and the same prediction algorithm is used irrespective of the DNA source, i.e. it does not require any adjustments or offsets.[1] This property allows one to compare the ages of different areas of the human body using the same aging clock.
Accuracy of the epigenetic clock in different body parts and cell types
The median error of predicted age is 3.6 years across a wide spectrum of tissues and cell types .[1] The epigenetic clock performs well in heterogeneous tissues (for example, whole blood, peripheral blood mononuclear cells, cerebellar samples, occipital cortex, buccal epithelium, colon, adipose, kidney, liver, lung, saliva, uterine cervix, epidermis, muscle) as well as in individual cell types such as CD4 T cells, CD14 monocytes, glial cells, neurons, immortalized B cells, mesenchymal stromal cells.[1] However, accuracy depends to some extent on the source of the DNA. In particular, DNAm age is higher than chronological age in female breast tissue that is adjacent to breast cancer tissue.[1] Since normal tissue, which is adjacent to other cancer types, does not exhibit a similar age acceleration effect, this finding suggests that normal female breast tissue ages faster than other parts of the body.[1]
Comparison with other biological clocks
The epigenetic clock leads to an age prediction that has a Pearson correlation coefficient of r=0.96 with chronological age (Figure 2 in [1]). Thus the age correlation is close to its maximum possible correlation value of 1. Other biological clocks are based on a) telomere length and b) p16INK4a expression levels (also known as INK4a/ARF locus).[16] The correlation between chronological age and telomere length is r=−0.51 in women and r=−0.55 in men.[17] The correlation between chronological age and expression levels of p16INK4a in T cells is r=0.56.[18] p16INK4a expression levels only relate to age in T cells, a type of white blood cells.
Age acceleration measure based on the epigenetic clock
By contrasting DNA methylation age (predicted age) with chronological age, one can define measures of age acceleration. Age acceleration, defined as difference between DNA methylation age and chronological age, is highly heritable. The broad sense heritability (defined via Falconer's formula) of age acceleration in older subjects is around 40% and it is 100% in newborns.[1]
Age acceleration in cancer tissue
Cancer tissues show both positive and negative age acceleration effects. For most tumor types, no significant relationship can be observed between age acceleration and tumor morphology (grade/stage).[1] On average, cancer tissues with mutated TP53 have a lower age acceleration than those without it.[1] Further, cancer tissues with high age acceleration tend to have fewer somatic mutations than those with low age acceleration.[1] Age acceleration is highly related to various genomic aberrations in cancer tissues. Somatic mutations in estrogen receptors or progesterone receptors are associated with accelerated DNAm age in breast cancer.[1] Colorectal cancer samples with a BRAF (V600E) mutation or promoter hypermethylation of the mismatch repair gene MLH1 are associated with an increased age acceleration.[1] Age acceleration in glioblastoma multiforme samples is highly significantly associated with certain mutations in H3F3A.[1]
Age acceleration due to obesity
The epigenetic clock was used to study the relationship between high body mass index (BMI) and the DNA methylation ages of human blood, liver, muscle and adipose tissue.[19] A significant correlation (r=0.42) between BMI and epigenetic age acceleration could only be observed for liver.
Age acceleration due to trisomy 21 (Down syndrome)
Down Syndrome (DS) entails an increased risk of many chronic diseases that are typically associated with older age. The clinical manifestations of accelerated aging suggest that trisomy 21 increases the biological age of tissues, but molecular evidence for this hypothesis has been sparse. According to the epigenetic clock, trisomy 21 significantly increases the age of blood and brain tissue (on average by 6.6 years).[20]
Relationship to a biological process that causes aging
It is not yet known what exactly is measured by DNA methylation age. Horvath hypothesized that DNA methylation age measures the cumulative effect of an epigenetic maintenance system but details are unknown. The fact that DNA methylation age of blood predicts all-cause mortality in later life [4] suggests that it relates to a process that causes aging.
Statistical approach for predicting age
The basic approach is to form a weighted average of the 353 clock CpGs, which is then transformed to DNAm age using a calibration function. The calibration function reveals that the epigenetic clock has a high tick rate until adulthood, after which it slows to a constant tick rate. Using the training data sets, Horvath used a penalized regression model (elastic net) to regress a calibrated version of chronological age on 21,369 CpG probes that were present both on the Illumina 450K and 27K platform and had fewer than 10 missing values. DNAm age is defined as predicted age. The elastic net predictor automatically selected 353 CpGs. 193 of the 353 CpGs correlate positively with age while the remaining 160 CpGs correlate negatively with age. R software and a freely available web based tool can be found at the following webpage.[21]
Other age predictors based on DNA methylation levels
Several other age predictors have been described in the literature.
1) Weidner et al. (2014) describe an age predictor for DNA from blood that uses only three CpG sites of genes hardly affected by aging (cg25809905 in integrin, alpha 2b (ITGA2B); cg02228185 in aspartoacylase (ASPA) and cg17861230 in phosphodiesterase 4C, cAMP specific (PDE4C)).[22] The age predictor by Weidener et al. (2014) only applies to blood. Even in blood this sparse predictor is far less accurate than the epigenetic clock (Horvath 2014) when applied to data generated by the Illumina 27K or 450K platforms. [23] But the sparse predictor was developed for pyrosequencing data and is highly cost effective. [24]
2) Hannum et al. (2013) [15] report several age predictors: one for each tissue type. Each of these predictors requires covariate information (e.g. gender, body mass index, batch). The authors mention that each tissue led to a clear linear offset (intercept and slope). Therefore, the authors had to adjust the blood based age predictor for each tissue type using a linear model. When the Hannum predictor is applied to other tissues, it leads to a high error (due to poor calibration) as can be seen from Figure 4A in Hannum et al. (2013). Hannum et al. adjusted their blood based age predictor (by adjusting the slope and the intercept term) in order to apply it to other tissue types. Since this adjustment step removes differences between tissue, the blood based predictor from Hannum et al. cannot be used to compare the ages of different tissues/organs. In contrast, a salient characteristic of the epigenetic clock is that one does not have to carry out such a calibration step:[1] it always uses the same CpGs and the same coefficient values. Therefore, the epigenetic clock can be used to compare the ages of different tissues/cells/organs from the same individual. While the age predictors from Hannum et al. cannot be used to compare the ages of different normal tissues, they can be used to compare the age of a cancerous tissue with that of a corresponding normal (non-cancerous) tissue. Hannum et reported pronounced age acceleration effects in all cancers. In contrast, the epigenetic clock [25] reveals that some cancer types (e.g. triple negative breast cancers or uterine corpus endometrial carcinoma) exhibit negative age acceleration, i.e. cancer tissue can be much younger than expected. An important difference relates to additional covariates. Hannum's age predictors make use of covariates such as gender, body mass index, diabetes status, ethnicity, and batch. Since new data involve different batches, one cannot apply it directly to new data. However, the authors present coefficient values for their CpGs in Supplementary Tables which can be used to define an aggregate measure that tends to be strongly correlated with chronological age but may be poorly calibrated (i.e. lead to high errors).
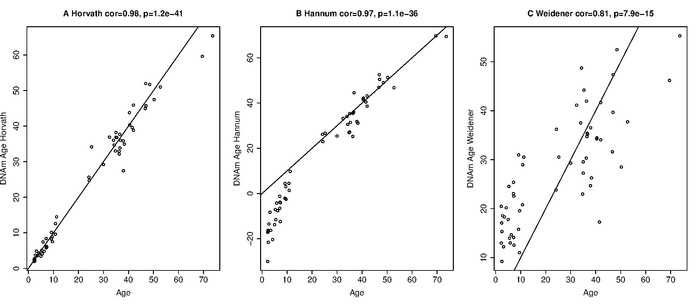
See also
References
- ↑ 1.0 1.1 1.2 1.3 1.4 1.5 1.6 1.7 1.8 1.9 1.10 1.11 1.12 1.13 1.14 1.15 1.16 Horvath S (2013) DNA methylation age of human tissues and cell types. Genome Biology.2013, 14:R115. DOI: 10.1186/10.1186/gb-2013-14-10-r115 PMID 24138928
- ↑ 2.0 2.1 University of California, Los Angeles (UCLA), Health Sciences (20 October 2013). "Scientist uncovers internal clock able to measure age of most human tissues; Women's breast tissue ages faster than rest of body". ScienceDaily. Retrieved 22 October 2013.
- ↑ University of California, Los Angeles (UCLA), Health Sciences (21 October 2013). "Scientists discover new biological clock with age-measuring potential". Forbes. Retrieved 21 October 2013.
- ↑ 4.0 4.1 Marioni R, Shah S, McRae A, Chen B, Colicino E, Harris S, Gibson J, Henders A, Redmond P, Cox S, Pattie A, Corley J, Murphy L, Martin N, Montgomery G, Feinberg A, Fallin M, Multhaup M, Jaffe A, Joehanes R, Schwartz J, Just A, Lunetta K, Murabito JM, Starr J, Horvath S, Baccarelli A, Levy D, Visscher P, Wray N, Deary I (2015) DNA methylation age of blood predicts all-cause mortality in later life. Genome Biology 16:25 doi:10.1186/s13059-015-0584-6
- ↑ Marioni R, Shah S, McRae A, Ritchie S, Muniz-Terrera GH, SE, Gibson J, Redmond P, SR C, Pattie A, Corley J, Taylor A, Murphy L, Starr J, Horvath S, Visscher P, Wray N, Deary I (2015) The epigenetic clock is correlated with physical and cognitive fitness in the Lothian Birth Cohort 1936. Int J of Epidemiology. Jan 22. pii: dyu277 PMID 25617346
- ↑ Biome on 21st October 2013 Novel epigenetic clock predicts tissue age
- ↑ Gibbs WT (2014) Biomarkers and ageing: The clock-watcher. Nature 508, 168–170 (10 April 2014) doi:10.1038/508168a
- ↑ Berdyshev G, Korotaev G, Boiarskikh G, Vaniushin B: Nucleotide composition of DNA and RNA from somatic tissues of humpback and its changes during spawning. Biokhimiia 1967, 31:88-993.
- ↑ Rakyan VK, Down TA, Maslau S, Andrew T, Yang TP, Beyan H, Whittaker P, McCann OT, Finer S, Valdes AM, Leslie RD, Deloukas P, Spector TD: Human aging-associated DNA hypermethylation occurs preferentially at bivalent chromatin domains. Genome Res 2010, 20:434-439
- ↑ Teschendorff AE, Menon U, Gentry-Maharaj A, Ramus SJ, Weisenberger DJ, Shen H, Campan M, Noushmehr H, Bell CG, Maxwell AP, Savage DA, Mueller-Holzner E, Marth C, Kocjan G, Gayther SA, Jones A, Beck S, Wagner W, Laird PW, Jacobs IJ, Widschwendter M (2010) Age-dependent DNA methylation of genes that are suppressed in stem cells is a hallmark of cancer. Genome Res 2010, 20:440-446.
- ↑ Koch CM, Wagner W 2011 Epigenetic-aging-signature to determine age in different tissues. Aging (Albany NY). 2011 Oct;3(10):1018-27.
- ↑ Horvath S, Zhang Y, Langfelder P, Kahn R, Boks M, van Eijk K, van den Berg L, Ophoff RA: Aging effects on DNA methylation modules in human brain and blood tissue. Genome Biol 2012, 13:R97 PMID 23034122
- ↑ Bell JT, Tsai PC, Yang TP, Pidsley R, Nisbet J, Glass D, Mangino M, Zhai G, Zhang F, Valdes A, Shin SY, Dempster EL, Murray RM, Grundberg E, Hedman AK, Nica A, Small KS, Dermitzakis ET, McCarthy MI, Mill J, Spector TD, Deloukas P, MuTHER Consortium: Epigenome-wide scans identify differentially methylated regions for age and age-related phenotypes in a healthy ageing population. PLoS Genet 2012, 8:e1002629
- ↑ Bocklandt S, Lin W, Sehl ME, Sánchez FJ, Sinsheimer JS, Horvath S, Vilain E (2011) Epigenetic Predictor of Age. PLoS ONE 6(6): e14821. doi:10.1371 PMID 21731603
- ↑ 15.0 15.1 Hannum G, Guinney J, Zhao L, Zhang L, Hughes G, Sadda S, Klotzle B, Bibikova M, Fan JB, Gao Y, Deconde R, Chen M, Rajapakse I, Friend S, Ideker T, Zhang K: Genome-wide methylation profiles reveal quantitative views of human aging rates. Mol Cell 2013, 49:359-367
- ↑ Collado M, Blasco MA, Serrano M. Cellular senescence in cancer and aging. Cell. 2007 Jul 27;130(2):223-33.
- ↑ Nordfjäll K, Svenson U, Norrback KF, Adolfsson R, Roos G (2010) Large-scale parent-child comparison confirms a strong paternal influence on telomere length. Eur J Hum Genet. 2010 Mar;18(3):385-9. doi: 10.1038/ejhg.2009.178 PMID 19826452 PMCID: PMC2987222
- ↑ Wang Y, Zang X, Wang Y, Chen P (2012) High expression of p16INK4a and low expression of Bmi1 are associated with endothelial cellular senescence in the human cornea. Molecular Vision 2012; 18:803-815.
- ↑ Horvath S, Erhart W, Brosch M, Ammerpohl O, von Schoenfels W, Ahrens M, Heits N, Bell JT, Tsai PC, Spector TD, Deloukas P, Siebert R, Sipos B, Becker T, Roecken C, Schafmayer C, Hampe J (2014) Obesity accelerates epigenetic aging of human liver. Proc Natl Acad Sci U S A . pii: 201412759. doi: 10.1073/pnas.1412759111 PMID 25313081
- ↑ Horvath S, Garagnani P, Bacalini MG, Pirazzini C, Salvioli S, Gentilini D, Di Blasio AM, Giuliani C, Tung S, Vinters HV, Franceschi C (2015) Accelerated epigenetic aging in Down syndrome. Aging Cell. 2015 Feb 9. doi: 10.1111/acel.12325 PMID 25678027
- ↑ DNA methylation age software
- ↑ Weidner, C. I., Lin, Q., Koch, C. M., Eisele, L., Beier, F., Ziegler, P., ... & Wagner, W. (2014). Aging of blood can be tracked by DNA methylation changes at just three CpG sites. Genome Biology, 15(2), R24.doi:10.1186/gb-2014-15-2-r24
- ↑ Horvath S (2014-02-18 16:34) Comparison with the epigenetic clock (2014). Reader Comment.
- ↑ Wagner W (2014) Response to comment "comparison with the epigenetic clock by Horvath 2013"
- ↑ Horvath S (2013-11-04 11:00) Erratum in cancer tissues Reader Comment